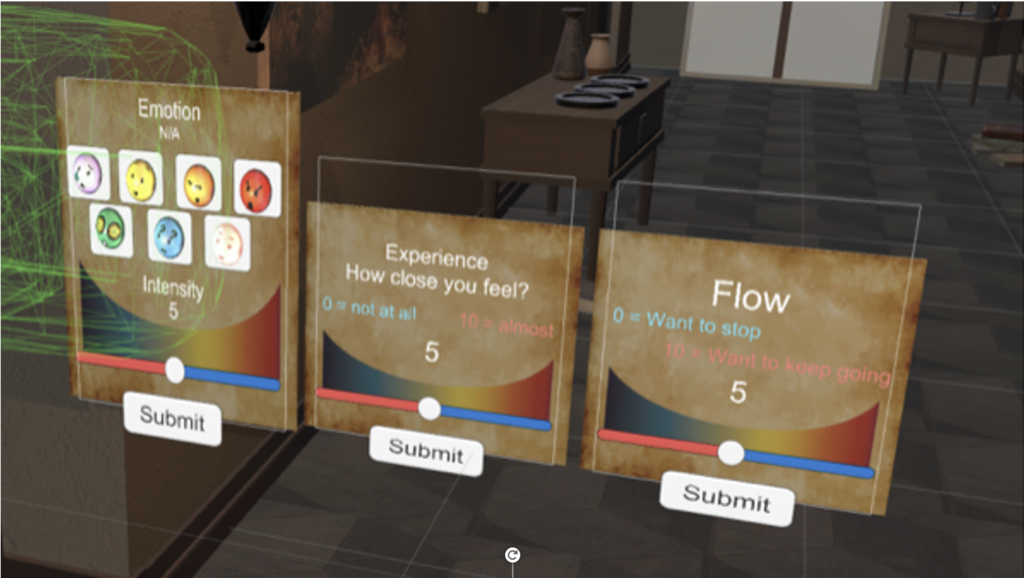
Non-Stationary Brain Network Dynamics
Ying Wu
September 16, 2019
Segmenting high dimensional physiological or behavioral data during Escape Room problem solving can be challenge. Here, we propose to track changes in non-stationary brain network dynamics. While working to solve Escape Room puzzles, participants log their motivational state (happy, lost, ambivalent, angry, sad, thinking, curious), how close they feel to solving the puzzle, and how much they would like to continue searching at five-minute intervals by means of an in-game survey that appears in the virtual environment (see image above). Their responses are time-stamped and synchronized with the concurrently recorded EEG.
After preprocessing and artifact correction or removal, the complete EEG data set undergoes Adaptive Mixture Independent Component Analysis (AMICA), developed by Jason Palmer at the Swartz Center for Computational Neuroscience, and described in Hsu et al (2018) (source below). AMICA is a general unsupervised-learning approach that uses a mixture of distinct ICA models – each representing a different set of statistically independent sources – to characterize underlying EEG source activities associated with distinct patterns of whole brain engagement. This approach allows the decomposition of the moment-to-moment fluctuations of activation of different brain systems from continuous, unlabeled EEG data during exploration of Escape Rooms. We will explore how different brain systems may come to be engaged at different intensities as a function of changes in motivational state recorded through the in-game surveys.
Hsu, S.-H., Pion-Tonachini, L., Palmer, J., Miyakoshi, M., Makeig, S., & Jung, T.-P. (2018). Modeling brain dynamic state changes with adaptive mixture independent component analysis. Neuroimage, 183, 47–61. doi:10.1016/j.neuroimage.2018.08.001